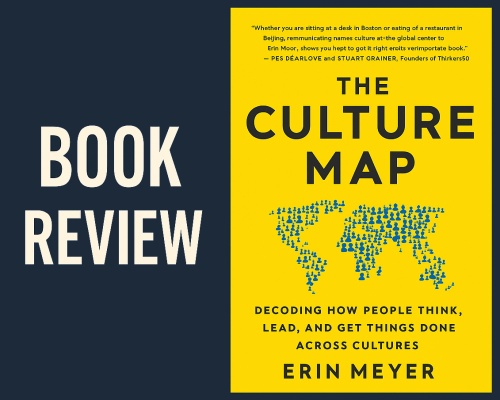
What "The Culture Map" Taught Me About Cross-Cultural Work and Trust
31.03.2025
Milo felt stuck again, and since the last time he spoke to fraud department colleagues, he was able to improve the model; he decided to try his luck again and talk to them once more. When he met them, he told them about his recent model improvement and that he couldn't see any other way to improve the model.
After listening to Milo, one of the fraud analysts noticed that Milo's model assessed the transactions as individual and independent; no memory was used to look at what was happening before this transaction. Analysts explained how some of their business rules look for sequences of transactions and how multiple transactions within a short time are simple examples of high-risk events.
This was an entirely new way of looking at
the problem. So he extended his ABT in his next revision cycle and added
additional columns – "number of transactions within last four hours",
"time since the last transaction," and a few more. He felt that this
might be a game-changer. But to calculate these details, he had to look at
every transaction in his ABT individually and consider the transactions before
that moment within the predefined time window. This wasn't a trivial task for
him as a data scientist, and he asked for help and advice from his data
management colleagues in IT who were more proficient in ETL building. With
their help, he prepared the columns. As he expected, the results were really
good. Within the subsequent few iterations, he played with different time
windows to get the best fit of the model.
After a few days, Milo felt that he had plateaued and that further fine-tuning the time windows and trying different sequences would bring no significant gains. He enthusiastically went to see the fraud risk colleagues again.
During this discussion, the fraud analyst
realized that Milo might have left early the last time as he forgot to tell him
about non-financial transactions. How extremely beneficial those might be in
the same context of using them as part of sequences. He also mentioned a couple
of examples: a change of mobile number shortly before funds transfer or
activation of the new channel before using it for funds transfer. Both patterns
significantly increase the fraud risk. Milo realized that he wasn't done with
the sequences of transactions yet, but he also remembered how he needed the help
of data management colleagues to get the code for calculating these extra
columns done.
Another problem that lurked right behind the corner was getting the non-financial transactions. So Milo went to talk to his data management colleagues again to figure out how big of a problem he was facing now. He briefly explained his high-level objective of building a predictive model for fraud detection and how he wants to include non-financial transactions into the mix. Though his colleagues were interested in the topics and the meeting went far beyond the scheduled time, he only shared high-level information without any precise details as his colleagues from the fraud risk team guided him.
The outcome of the discussion was not a
complete disaster, but it wasn't a clear victory either. For example, some
non-financial transactions (like a change of mobile number, change of address, or
request to issue a new checkbook) were available in the DWH in a specific table.
But other events like "successful login" or "failed login"
were not available as the channel itself managed those events. Colleagues also
told him that they could potentially bring those events (and others) into the
DWH, but till that moment, there was no particular use case for such data.
Still, the difficult part would be to transform the non-financial events into a meaningful flag like "was there a change of mobile number within 24h" or "was there a registration of a new trusted device within 24h". But the colleagues from the data management team came to the rescue and helped Milo prepare the codes to derive the required details.
Another round of training the model and validating the results brought positive news, and the model's accuracy improved again. Looking back at all the different data that ABT was working with, Milo was quite impressed. Yet he wanted to know if there was more that could be done to supply more details that could turn out to be better fraud indicators than the ones already identified. So, without any hesitation, he went back to his fraud-risk colleagues to brainstorm potential new ideas.
After sharing his latest adjustments and their contribution to the accuracy, they decided to jointly discuss the last few fraud incidents and analyze the pattern behind them to see whether some new approach could be identified.
While going through the details, they could see that most of the frauds in this typology are linked to social engineering techniques and how they often end up as account takeover frauds. Fraud analysts mentioned how they had deployed rules looking for the customer's unusual behavior. They checked whether customers' present amounts and volumes of transactions are not uncommon (too high), whether the channel is used in alignment with previous observations, and some other patterns. To Milo, it was apparent that this could potentially improve the accuracy, but it would surely lower the false positives, as the model would be able to spot abnormal or anomalous customer behavior more accurately.
"So what would it mean to build such
profiles?" Milo asked himself. Thinking about it, he realized that he
might need additional data, even before 6months, if he wanted to build a
profile for all transactions within his ABT. For example, suppose he wanted to
calculate the "maximum of the cumulative daily amount of debits for the
given customer within last month" for today's transaction. In that
case, he will need the previous month's transactions, calculate the cumulative
daily amount of debits for each customer for each day and then select the
maximum from these 30 days for each customer. But for the transactions from
6 months back, he would need the data from 1 month prior - to the 7th
month. Extending the data based on the depth of the historical window was the
first complication.
Each characteristic is linked to a particular
entity – most commonly a customer. So, for example, we can calculate the number
of transactions the customer has done in 24h; similarly, we can calculate the
statistics on the account level – the number of transactions in 24h for each
account independently or each channel alone. We can also calculate other metrics
– minimum, average, percentiles, etc. For example, we can sum amounts or counts daily,
weekly, and monthly; we can consider any transaction or be very narrow and count
only debit transactions performed via a branch to a particular country, etc.
Other entities could be an employee or product like a credit card, debit card,
checkbook, etc.
The options were limitless, and only now Milo realized how important it is to understand the fraud schemes to be able to design and calculate new columns, which would eventually become part of the model and help distinguish the frauds from the genuine transactions.
Milo knew that his visit to fraud risk
colleagues was just the beginning, and to improve the model further, he would
need to gather as many insights as possible.
When he was reading about different
detection techniques – especially the ones related to payment fraud perpetrated
through e-channels – he found an article that mentioned a new term –
device fingerprinting. Milo was surprised how some scripts were able to capture
different static as well as behavioral characteristics. While the web visitor
was browsing through the pages, the script would capture static details like - device
type (PC, iOS, Mac, Android), device language settings, screen resolution,
firmware version, timezone, IP, browser details, and many others. It was also
possible to capture specific behavioral characteristics like typing speed, mouse
cursor movement, duration of visit to each page, and others.
If these details were available and could
be added to the ABT, they would surely further improve the accuracy and lower
the false positives. But this would be something that Milo will need to discuss
with a broader audience within the bank as integrating external 3rd party data
would require a project of its own.
Milo closed the browser with mixed feelings
– realizing that his journey to enhance the model further would never end. Even
though he felt he had reached the end many iterations back, it was clear
that the actual end was nowhere near his reach.
Was Milo successful in deploying his model
into production? Did he make any mistake that will cause trouble while Milo
tries to operationalize the model?
Disclaimer: The
story, all names, characters, and incidents portrayed in this article are
fictitious. No identification with actual persons (living or deceased), places,
buildings, and products is intended or should be inferred.